Strategies for Achieving Artificial General Intelligence
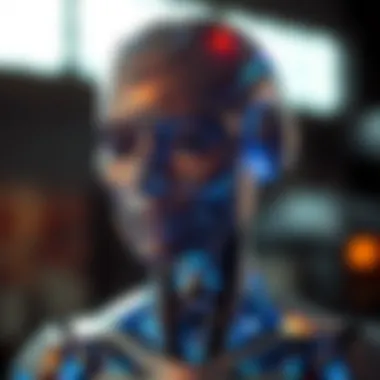
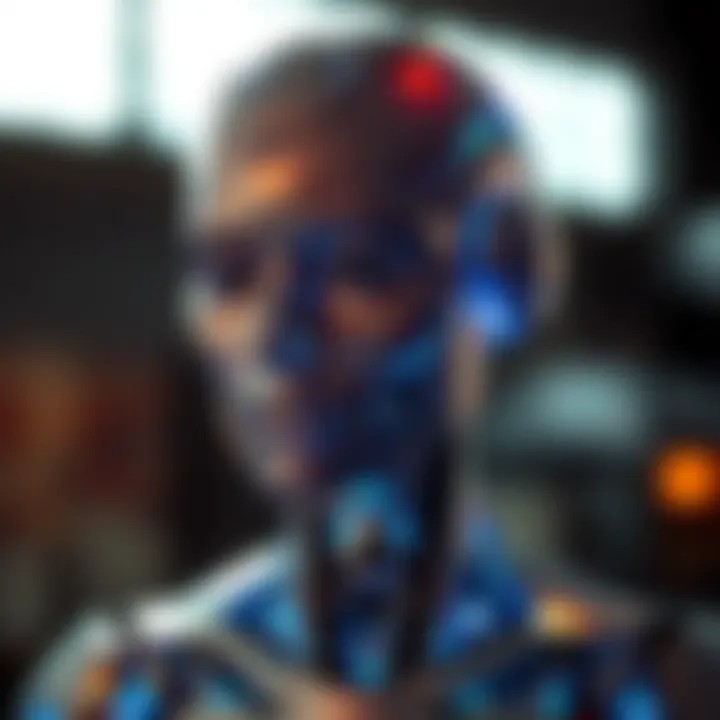
Intro
In the field of artificial intelligence, discussions around achieving Artificial General Intelligence (AGI) have surged in recent years. Unlike narrow AI, which excels in specific tasks, AGI embodies the ability to learn, reason, and understand across a broad array of domains ā akin to a human mind. This quest for AGI is not merely an academic endeavor; it holds profound implications for industries, societies, and ethical frameworks. As we embark on this exploration, it's essential to understand the strategies that could pave the way for AGI and the unique challenges that lie ahead.
AGI is often painted as a futuristic concept, but the steps toward realizing it are firmly rooted in today's technological landscape. The focus must include interdisciplinary collaboration, advanced algorithm design, and a firm commitment to ethical considerations. Examining these aspects can help individuals and organizations navigate this intricate terrain.
This article seeks to break down these strategies into digestible parts, guiding readers through various methodologies, technological advancements, and ethical implications connected to AGI. Let's deepen our understanding of the approaches fueling this fascinating journey.
Understanding Artificial General Intelligence
Artificial General Intelligence (AGI) sits at the intersection of aspiration and reality in the field of artificial intelligence. This concept embodies the pursuit of creating machines that not only simulate human reasoning but also possess the ability to understand and learn any intellectual task that a human can. Delving into AGI isnāt just an academic exercise; itās a point of convergence for engineers, scientists, and philosophers alike, holding immense implications for the future of technology and humanity itself.
Defining AGI
Defining AGI starts with understanding it as more than a tool; it is, in essence, a system capable of independent thought, adaptation, and learning across a myriad of domains. Unlike specialized AIāsystems designed for limited tasks, like recommending moviesāAGI aims for broad-spectrum reasoning and comprehension. Itās essential to establish this definition to frame the conversation about its potential applications and implications.
What distinguishes AGI?
AGI is characterized by its autonomous cognitive abilities, contrasting with narrower systems that excel only in predefined functions. Consider how a human learns: empathy, reasoning, and complex problem-solving are all innate capabilities that AGI theorists strive to replicate in machines.
Distinguishing AGI from Narrow AI
To illuminate the differences between AGI and Narrow AI, it helps to think of Narrow AI as the current bread and butter of AI technology. These systems excel in specific tasksālike voice recognition or data processingābut lack understanding or generality. For instance, a voice assistant can answer your questions but canāt comprehend the intricacies of human emotions.
AGI, in contrast, would be like a universal translator of sorts, understanding intention and context, and consequently adapting its responses to fit a range of situations. This distinction is crucial not just for categorizing existing technologies but also for setting the groundwork for future innovations. In a world stocked with specialized algorithms, the leap to AGI remains a grand ambition.
Historical Context
The quest for AGI is anything but recent; it stretches back to the early days of computer science. From Alan Turingās foundational concepts in the 1950s to modern neural networks, the journey has been fraught with enthusiasm as well as skepticism. Researchers have cycled through periods of optimism and disillusionment, shaped by technological limitations and philosophical debates surrounding consciousness and cognition.
- In the 1960s and 70s, developments in symbolic AI prompted excitement but soon met obstacles, mainly due to limited computational power.
- The 1990s, however, ignited renewed interest in machine learning, culminating in various approaches designed to mimic human available learning models.
"To understand AGI, one must appreciate not just technology but also the philosophical questions it raises about the nature of intelligence and consciousness."
In revisiting historical milestones, we grasp the context that defines current AGI pursuits. The rhythm of our understanding has been profoundly influenced by previous insights, mistakes, and breakthroughs. This historical lens frames the present discussion on AGI, reminding us that while technology moves rapidly, the questions surrounding it remain complex and multifaceted.
In exploring these foundational elements, we pave the way for recognizing how AGI can redefine not merely technology but also human potential.
Core Principles of AGI Development
Core principles in AGI development serve as the backbone for pursuing true intelligence that can parallel or exceed human cognitive functions. Understanding these principles is essential for researchers, developers, and investors alike, as they illuminate the pathways and methodologies that can make AGI not just a distant dream but an achievable reality.
A thorough grasp of these core principles offers several benefits. For one, it clarifies how different components interact. This foundational knowledge is integral for those venturing into the complex world of AGI. Furthermore, it helps academics, engineers, and enthusiasts in aligning their tools, models, and perspectives towards a unified goal.
Cognitive Architectures
Cognitive architectures are structured frameworks that outline how an AGI system processes information, learns from experiences, and utilizes knowledge. Picture a blueprint for intelligence; it provides a clear overview of how cognitive processes operate in tandem. This can include everything from perception and memory to decision-making and reasoning. A compelling architecture offers an effective starting point for simulating human-like understanding.
For instance, consider the SOAR architecture, which emphasizes the importance of both symbolic and sub-symbolic processing. Through SOAR, AGI systems can harness various types of knowledge, making them more adaptable to different situations.
Moreover, incorporating cognitive architectures enables software to simulate emotional and social interactions, which can be crucial for debugging and fine-tuning AGI systems. The seamless integration of these processes can directly contribute to a more holistic approach in developing AGI.
Learning Algorithms
Learning algorithms are the mental faculty, so to speak, for AGI, akin to how humans learn from their environment. These algorithms determine how an AGI system adjusts its behavior based on experiences. They fall into several categoriesāsupervised, unsupervised, reinforcement learningāall of which play vital roles in how intelligence is acquired and refined.
Take, for example, Deep Reinforcement Learning (DRL). This method not only teaches an AGI system to make decisions but also allows it to explore and exploit its environment effectively. Thanks to DRL, AI has made leaps in mastering complex games, from chess to video games. The implications stretch beyond games and touch everything from robotics to autonomous driving. The more sophisticated the learning algorithms, the more nuanced and effective the AGI will become.
Knowledge Representation
Knowledge representation relates to how information is stored and utilized within an AGI system. It encompasses the semantics, reasoning, and ontology that helps the AGI understand relationships between different pieces of knowledge. Representing knowledge effectively is crucial for enabling systems to draw inferences, generalize from past experiences, and understand context.
Consider knowledge graphs as one example of this principle at work. These graphs provide a visual and structured way to represent relationships between concepts, making it easier for AGI systems to navigate complex datasets. The effectiveness of an AGI ultimately depends on its ability to not just store knowledge, but to leverage it meaningfully, which can lead to insightful conclusions and decisions.
"The real challenge lies in not just accumulating knowledge, but understanding its context and applicability within various frameworks."
By weaving together cognitive architectures, learning algorithms, and knowledge representation, the pursuit of AGI becomes structured and feasible. Aspiring AGI researchers must prioritize these principles as foundational elements that can unlock the myriad possibilities that AGI presents.
Interdisciplinary Collaboration
Interdisciplinary collaboration stands at the forefront of efforts aimed at achieving Artificial General Intelligence (AGI). This necessity emerges from the multifaceted nature of AGI itself, which requires melding insights and methodologies from a variety of disciplines. To broaden the horizons of understanding and innovation in AGI, cooperation between fields such as neuroscience, psychology, and computer engineering becomes critical. Here, we will examine the various aspects of this collaboration, highlighting its benefits, challenges, and how it can lead to significant advancements in AGI development.
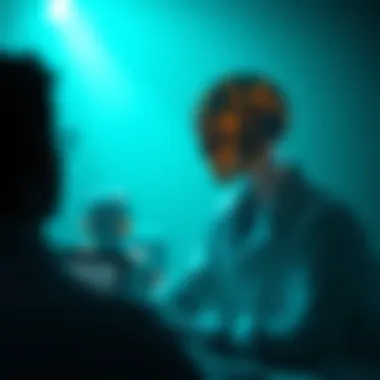
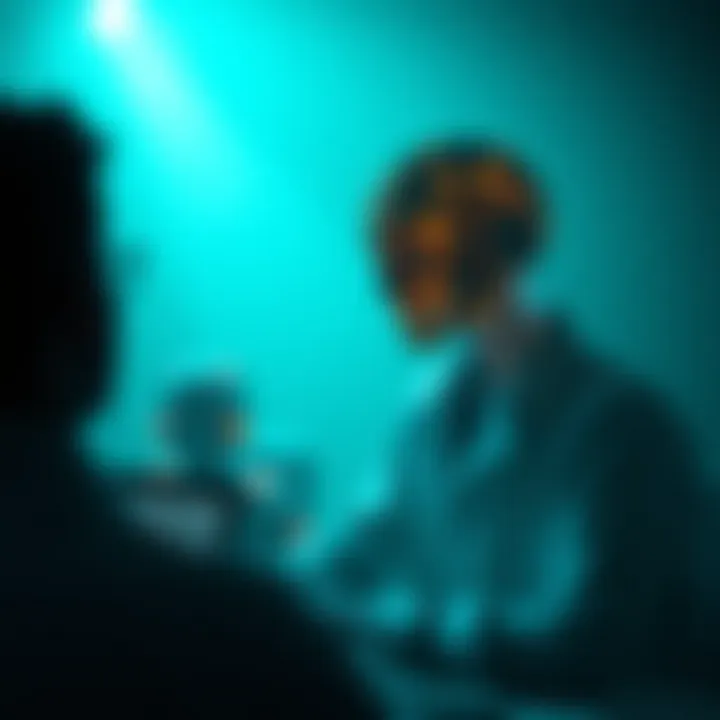
Incorporating Neuroscience
Understanding how the brain processes information is a pivotal element in the quest for AGI. Neuroscience unravels the intricacies of neural networks, cognitive functions, and the biological underpinnings of learning. By integrating findings from this field, researchers can gain insights into how to design algorithms that mimic these processes. The methodology of neural networks itself was inspired by concepts in neuroscience, showcasing the direct impact this discipline has on AGI technology.
Additionally, neuroscience offers valuable lessons on resilience and adaptability in learning. Human brains are not merely hardwired; they constantly reorganize based on experiences and environmental stimuli. This adaptability can inspire more sophisticated AGI systems, allowing them to learn from failure just as humans do. The input from neuroscience can help develop models that emulate such flexibility, which is essential for achieving true general intelligence.
Role of Psychology
Psychology provides an essential understanding of cognition, emotion, and behaviorāfactors that are often overlooked in traditional AI models. By examining how humans think, learn, and interact with the world, psychological principles can inform the design of AGI systems that operate more naturally and effectively in social contexts.
Human-like intelligence also includes elements of decision-making, moral reasoning, and emotional intelligence. Incorporating psychological insights can yield AGI that better understands human needs and societal norms. For instance, natural language processing can greatly benefit from psychological theories about discourse and social interaction. Furthermore, understanding the psychological aspects of bias helps in creating algorithms that can recognize and mitigate their own biases, a crucial step for ethical AGI development.
Engineering and Software Development
Developing AGI requires cutting-edge engineering and software development practices. Engineering acts as the bridge linking theoretical research to practical implementation. It ensures that frameworks drawn from neuroscience and psychology can be effectively encoded into functional AGI systems. Strongly interlinked, these disciplines work together to produce agile, maintainable, and scalable software solutions.
Incorporating modern software engineering practices like agile methodologies allows cross-disciplinary teams to iterate and improve upon designs quickly. This recipe for innovation, when mixed with engineering principles that emphasize modular architectures and robust testing protocols, vastly accelerates the exploration of AGI pathways.
To summarize, interdisciplinary collaboration serves as a cornerstone for achieving AGI. By uniting neuroscience, psychology, and engineering, the AI community can harness a comprehensive range of perspectives, enriching their research and potentially speeding up the realization of AGI. Each discipline contributes unique perspectives and tools, creating a fusion that can lead to more sophisticated and adaptable AGI systems.
Current Challenges in AGI Research
The pursuit of Artificial General Intelligence (AGI) is exciting, yet riddled with hurdles that researchers and practitioners must navigate. Understanding these challenges is crucial, as they shape the methodologies and frameworks we can develop. This section focuses on three primary obstacles: technical limitations, data privacy concerns, and ethical dilemmas. Each of these elements carries distinct implications for the trajectory of AGI development, offering insights that can inform both current practices and future innovations.
Technical Limitations
AGI aims to mimic human intelligence across various tasks. However, current AI technologies often struggle with flexibility and generality. While narrow AI has made significant stridesāthink of how Google's DeepMind plays chess or how Amazon's Alexa understands commandsāthese systems lack the broader understanding necessary for AGI. One major technical limitation is the ability to reason across diverse domains and contexts. Traditional machine learning algorithms typically operate on specific datasets and can fall short outside these narrow confines.
"To achieve AGI, we must extend beyond mere pattern recognition; we need systems that can comprehend the world as humans do."
Even more, the computational power required for developing AGI can be a double-edged sword. While larger datasets and more complex algorithms emerge, the cost and infrastructure needed to support this level of computation often stifles innovation. Moreover, the performance of neural networks plateaus as model sizes increase, suggesting a dire need for novel architectures that can capture the nuances of human-like thought.
- Potential strategies to overcome these limitations include:
- Exploring neuromorphic computing, which mimics the human brain's architecture.
- Developing hybrid architectures that combine symbolic reasoning with deep learning.
Data Privacy Concerns
As AGI systems require vast amounts of data for learning, data privacy stands as a prominent concern. The increasing awareness of privacy issues in digital platforms complicates the landscape for AGI research. Achieving AGI necessitates access to diverse datasets, often involving sensitive personal information. Collecting, storing, and utilizing these data while ensuring compliance with regulations such as GDPR or HIPAA becomes a major hurdle.
Many researchers advocate for privacy-preserving methods such as federated learning, where models learn from decentralized data sources without compromising individual privacy. The implementation of such methods, however, often introduces additional complexities. The fine balance between effective data utilization and user privacy will be crucial for the acceptance and ethical deployment of AGI systems.
- Considerations include:
- Transparent data usage policies
- User consent mechanisms
Ethical Dilemmas
The ethical landscape of AGI research presents a myriad of dilemmas that require careful consideration. As we develop systems that potentially rival human intelligence, questions about accountability arise. Who is responsible when an AGI system causes harm? The ambiguity surrounding this question poses a significant barrier.
Additionally, there is a pressing concern about biases present in data and algorithms. If AGI systems learn from flawed datasets, they may perpetuate or even amplify existing societal biases. This reality underscores the need for thorough vetting of both data and algorithms before deploying AGI technologies.
Furthermore, the implications of single entities controlling powerful AGI systems raise alarms about power dynamics, equity, and access.
- Essential ethical principles to consider include:
- Fairness in algorithm design
- Continuous monitoring for unintended consequences
In summary, while the journey toward AGI is fraught with challenges, addressing these barriers head-on is crucial. Technical limitations, data privacy concerns, and ethical dilemmas not only require innovative solutions but also compel researchers to rethink traditional approaches. Engaging in a discourse around these issues will not only augment the development of AGI but also ensure that it aligns with societal values and norms.
Exploring Pathways to AGI
Exploring pathways to Artificial General Intelligence (AGI) is crucial for several reasons. First off, it lays down the roadmap for researchers and developers aiming to create machines that think like humans. The exploration process highlights various strategies and methodologies that can be instrumental in overcoming the monumental challenges faced in AGI development. Each pathway offers distinct advantages, ultimately contributing to the common goal of building a more intelligent and adaptable AI.
Another vital element in this exploration is the interplay between theory and practice. For every theoretical framework, there exists a set of practical implications that can dictate the success of AGI technologies. As researchers consider these pathways, itās essential to weigh the benefits against potential blockers, such as resource allocation, ethical dilemmas, and technological feasibility.
Additionally, diving into these pathways encourages interdisciplinary collaboration, as insights from fields like neuroscience, psychology, and computer science converge. This melding of expertise is where innovative ideas can truly flourish. By unpacking the various options available, we gain a clearer view of the complex landscape of AGI and identify actionable steps towards realization.
Incremental Learning Approaches
Incremental learning approaches focus on the idea that AGI systems can learn gradually from new data instead of needing to retrain from scratch. This kind of learning mirrors human cognitive processes, where knowledge builds over time through experience. Researchers are beginning to see the merits of these methodologies, particularly in dealing with vast amounts of dynamic data that typical AI systems struggle with.
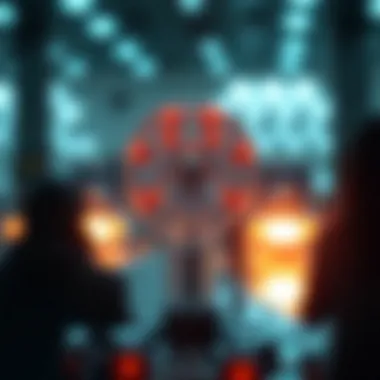
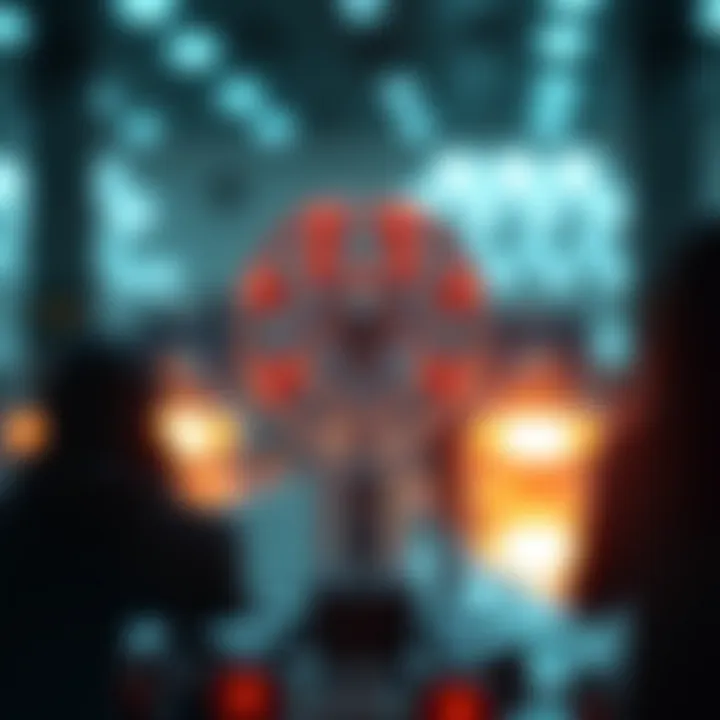
An example of incremental learning can be seen in algorithms that adapt to user input without forgetting past information. This is a critical requirement for AGI systems, which need to retain knowledge while also evolving. Consider the application of reinforcement learning, where an AGI model improves its actions based on feedback. This method encourages continuous improvement and fine-tuning, ensuring that the system becomes increasingly adept over time.
Incremental learning isn't just a theory; it's rapidly becoming a necessity as data grows exponentially in todayās digital landscape.
Simulations and Virtual Environments
Simulations and virtual environments are increasingly becoming valuable tools in the pursuit of AGI. These settings allow researchers to replicate complex real-world scenarios without the associated risks. The beauty of a simulation is its flexibility; researchers can tweak variables, alter environments, and observe interactions in ways that wouldnāt be feasible in reality.
For instance, consider a virtual space designed to test an AGI's decision-making skills under different stress scenarios. Researchers can simulate various situationsāfrom a high-traffic city to a flight control systemāallowing the AGI to make decisions based on perceived conditions. Over time, these simulations help refine the AGIās responses, making it more robust and adaptable.
Moreover, these environments help in the training process, giving AGI systems the capacity to evaluate their actions and outcomes in a safe context. This can lead to significant advancements in emotional intelligence within AGI systems, as they learn to navigate social cues and human interactions more naturally.
Human-AGI Collaboration
The collaboration between humans and AGI systems is an area that holds remarkable potential for future innovations. The idea here is not to replace human intelligence but to enhance it. By working alongside these intelligent systems, humans can benefit from optimized decision-making, greater efficiency, and improved creativity.
In practical terms, think of tools like Google Docs that offer smart suggestions for grammar or structure as one simple example. These incremental assistants illustrate how AGI can play a complementary role in our daily tasks. Furthermore, partnerships in industries like healthcare show promising results; AGI systems can analyze patient data more swiftly than a human can and provide insights that practitioners can use.
Ultimately, the synergy between human intelligence and AGI can lead to breakthroughs in areas such as natural language processing, predictive analytics, and personalized learning experiences. To harness this potential fully, both sides must share knowledge and adapt to one anotherās strengths and limitations.
The Role of Funding and Investment
When it comes to advancing Artificial General Intelligence (AGI), funding and investment play pivotal parts. The sheer complexity and ambition of AGI research demand significant financial resources. Whether itās the development of robust cognitive architectures or exploring multidisciplinary approaches, the monetary backing influences all phases of AGI progression. Without adequate funding, many promising theories and projects may remain mere sketches in a lab notebook, stifling innovation and practical application.
Public vs. Private Funding
The funding landscape for AGI is filled with nuances that can affect its overall trajectory. Public funding often comes from governmental sources, intended to foster scientific research and address societal challenges. This funding model enables researchers to tackle projects that may not yield immediate financial returns but have long-term benefits for humanity. For instance, initiatives like the Defense Advanced Research Projects Agency (DARPA) significantly contribute to AGI through both resource allocation and coordinated research efforts.
On the other end of the spectrum, private funding channelsāespecially from tech giants like Google and Microsoftābring agility and competitive spirit into the ring. Private firms can harness their profits for cutting-edge research, employing rapid prototyping methods with less bureaucratic red tape. Yet, they may prioritize projects that are more inclined toward profit rather than purely scientific endeavors. There's value in balancing these approaches:
- Public funding often lacks the agility of private investments but can provide stability and direction in research.
- Private investment can drive innovation quickly, though it might veer toward commercial interests over profound scientific inquiries.
Understanding the interplay between these two types creates a multifaceted funding environment suitable for AGI's unique demands.
Investment in Research Initiatives
Investment in AGI research initiatives signifies a commitment to not just understanding the technology, but applying it. Research bodies and universities require consistent funding to develop new algorithms, study human-computer interaction, and, importantly, engage in ethical discussions surrounding AGI. The National Science Foundation is one example that allocates funds to AGI research, often aiming to pull together scientists from various fields.
In addition to traditional research institutions, organizations promote research ventures focused on AGI breakthroughs:
- Nonprofits advocating for safe and ethical AI development.
- Collaborations between academia and industry draw expertise from diverse areas.
Collaboration also breeds innovation. For example, Googleās AI division often works hand-in-hand with academic partners, leveraging their insights while also giving back to the community through knowledge sharing and tools for education. A combined approach can maximize resources and talent across multiple domains.
Impact of Startups on AGI Progress
Startups are an often overlooked yet crucial part of the landscape in AGI research. Frequent pressure to innovate drives these smaller companies to respond quickly to the initial findings and bring new ideas to market at a breakneck speed. While they may not have the gigantic resources of established tech giants, they often possess focused expertise in niche areas of AGI research. This focus allows them to experiment without the burden of established corporate structures.
Many successful AGI approaches are born from startup innovation, leveraging venture capital to cultivate new ideas and methodologies. This agile environment encourages disruption and can sometimes lead to breakthroughs not anticipated by larger organizations. However, itās important to approach startup developments with a critical mind:
- Risk of burnout due to high ambition.
- Variability in project scopes leads to inconsistent results in research.
Nonetheless, as startup ecosystems flourish in various parts of the globe, their contributions to AGI development cannot be overstated.
In the world of AGI, funding alone doesnāt guarantee progress; the collaboration between diverse sourcesāpublic, private, and innovative disruptorsāis essential for shaping the future.
Foundational Knowledge for Aspiring AGI Researchers
Understanding the principles behind Artificial General Intelligence (AGI) is key for those who aspire to enter this complex field. This foundational knowledge serves as a firm base where theoretical understanding meets practical know-how. Grasping the core concepts not only empowers researchers but also shapes their approach to tackling the nuances and challenges inherent in AGI development. Essentially, this knowledge can act as a compass, directing individuals through the myriad of methodologies and theories essential for AGI research.
Educational Pathways
When it comes to education, aspiring researchers have a plethora of options available. Universities increasingly offer specialized degrees focusing on artificial intelligence, machine learning, and related fields. Here are some commonly pursued educational pathways:
- Bachelor's Degree in Computer Science or Engineering: A solid initial step where one develops programming skills and learns about algorithms.
- Master's Degree in AI or Robotics: This stage allows for deeper exploration of specialized topics, including neural networks and cognitive robotics.
- PhD Programs: Research-oriented programs enable individuals to conduct original research, pushing the boundaries of current knowledge.
Besides formal degrees, there are online courses available through platforms like Coursera, edX, and Udacity. These often cover specific topics like reinforcement learning and natural language processing, making them highly accessible.
Essential Skills Development
To excel in the AGI domain, gaining various skills is crucial. Knowledge alone wonāt cut it; hands-on experience combined with diverse skill sets prepares researchers for real-world challenges. Some critical skills include:
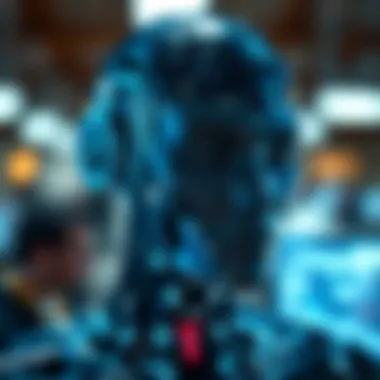
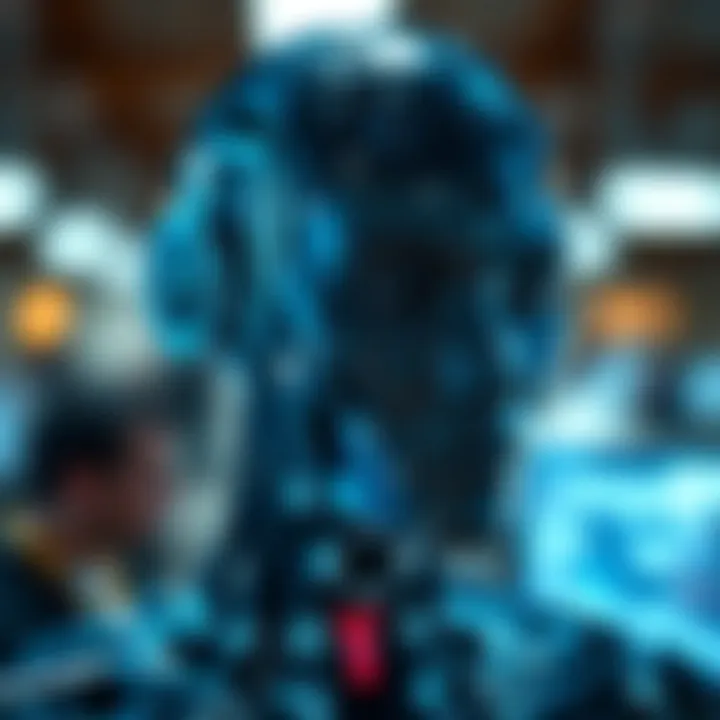
- Programming Languages: Proficiency in languages like Python and Java is essential, given their extensive use in AI projects.
- Data Analysis: Understanding how to analyze large datasets is vital for training AGI models effectively.
- Mathematics and Statistics: A strong grasp of statistical methods aids in algorithm development and performance evaluation.
- Problem-Solving Abilities: Researchers must think critically and creatively, navigating perplexing problems that arise during AGI development.
Building such a skill set requires commitment and an ongoing desire to learn and adapt.
Engaging with the Research Community
Finding a sense of belonging in the AGI research community can greatly enhance one's career potential. Active engagement not only opens doors to collaborations but also keeps researchers abreast of the latest developments. Here are ways to connect:
- Conferences and Workshops: Attending events like NeurIPS or ICML provides exposure to cutting-edge research and networking opportunities.
- Online Forums and Social Media: Platforms such as Reddit and Facebook have communities where researchers and enthusiasts share insights and resources.
- Publications and Journals: Contributing to or reading journals such as the Journal of Artificial Intelligence Research can deepen understanding and help researchers find their niche.
Engagement fosters a sense of community and shared knowledge, vital for anyone journeying into the intricate world of AGI.
"Knowledge without action is meaningless. Participation in the community is where ideas come to life."
In summation, foundational knowledge, education, skill development, and community engagement form the cornerstone for success in the pursuit of Artificial General Intelligence. With the right approach, aspiring researchers can navigate this complex realm effectively, paving the way for innovative breakthroughs.
Ethical Considerations of AGI
The discussion surrounding Artificial General Intelligence (AGI) is not just about technology; it delves into a realm that intertwines morality, accountability, and social responsibility. As AGI starts to emerge from the realms of theory into potential practice, the ethical implications of its development cannot be sidelined. Engaging robustly with these considerations is paramount for ensuring AGI technologies serve humanity positively, rather than spiraling into unchartered territories where their effects could be detrimental.
Understanding the nuances of these ethical considerations provides a framework for responsible AGI development. When implementing AGI systems, developers must grapple with questions like: What happens if an AGI system makes a decision that leads to harm? Who is held accountable in such cases? Establishing a baseline of accountability is crucial, as it shapes public trust and the broader societal acceptance of AGI.
Moreover, addressing ethical considerations promotes transparency in AGI systems. When potential flaws and biases are acknowledged upfront, it fosters dialogue among stakeholdersāranging from engineers and researchers to policymakers and the public. This willingness to engage openly supports an ecosystem conducive to innovative yet responsible AGI development.
Accountability in AGI Systems
Accountability within AGI systems is a pressing concern. In a world where machines are endowed with decision-making capabilities, understanding who is responsible for these decisions is vital. There are several intricacies involved:
- Human Oversight: AGI systems should not operate in a vacuum. Continuous human supervision is essential to ensure that decisions align with ethical standards and public expectations.
- Designing Fail-safes: Implementing mechanisms that can halt unjust decisions before they manifest in the real world is one potential safeguard.
- Clear Accountability Structures: Establishing clear roles and responsibilities can help delineate who is accountable in case of failures or adverse consequences. This clarity can shield developers and organizations from potential backlash while fostering a sense of ownership towards the systems they create.
"Accountability is the cornerstone of ethical AI implementation. Without it, we risk sacrificing trust at the altar of innovation."
Addressing Bias in Algorithms
Algorithms are the backbone of AGI systems, but they come with their own set of biases, which can lead to unjust outcomes. When developing AGI, it is essential to confront these biases head-on:
- Data Quality: The datasets used to train AGI systems often reflect societal biases. By scrutinizing and refining these datasets, researchers can reduce the risk of perpetuating discrimination.
- Bias Audits: Regular audits examining how a model performs across different demographics can identify underlying biases that need addressing.
- Diverse Development Teams: Engaging professionals from varied backgrounds can contribute unique perspectives, enabling more equitable algorithms that account for different experiences and viewpoints.
Long-term Implications of AGI
The long-term implications of AGI are profound and complex. As we tread into this future, several factors warrant our attention:
- Societal Impact: How AGI influences employment, ethical norms, and social interactions could reshape entire industries and cultures. We need to envisage a world where AGI augments human capabilities without rendering positions obsolete.
- Regulatory Frameworks: Developing robust regulations to oversee AGI implementations is essential to mitigate risks associated with misuse or unintended consequences.
- Evolution of Human-AI Relationships: As AGI systems become more integrated into daily life, understanding how humans relate to these systems will dictate how society as a whole navigates this new paradigm.
Future Trends in AGI
Understanding the future trends in Artificial General Intelligence (AGI) is crucial as it not only frames the trajectory of research but also highlights the pressing challenges and opportunities that lie ahead. As the landscape of technology evolves, so too will the methodologies and frameworks used to achieve AGI. This section examines how advancements can shape development, integration into society, and collaboration on an international scale. Recognizing these trends aids in preparing researchers, investors, and policymakers alike for the shifting tides of AGI.
Advancements in Computational Power
The backbone of any AGI initiative lies in its computational power. As technology progresses, we witness a rapid enhancement in hardware capabilitiesāthink of the leap from standard processors to the advent of quantum computing. Quantum computers have the potential to solve complex algorithms at unprecedented speeds, allowing AGI systems to learn at rates that could make todayās AI look like a child fumbling with building blocks. More processing power directly translates to more intricate models, capable of mimicking human cognitive functions in a more realistic manner.
- Benefits of Enhanced Power:
- Faster processing times, enabling near-real-time learning.*
- Ability to analyze vast amounts of data quickly, improving learning efficiency.*
- Support for advanced simulations that can mimic real-world interactions.
However, with great power comes great responsibility. Ethical frameworks must evolve concurrently to address potential misuses, such as invasive surveillance or deepfakes generated by exceptionally intelligent AGI systems. Fostering a dialogue on guidelines around computational power will be key.
Integration of AGI into Society
Integrating AGI into society extends beyond mere application; it heralds a transformative societal shift. The goal isnāt to replace human intelligence but rather to complement it. Consider areas like healthcare, where AGI could enhance diagnostics or personalized medicine, becoming a crucial ally for physicians. In education, personalized learning powered by AGI could adapt curricula to fit students' individual needs.
Benefits of this integration involve:
- Efficiency improvements in various sectors.
- Enhanced decision-making capabilities in business through data analysis.
- Increased accessibility, particularly for individuals with disabilities, as AGI-powered tools can provide tailored assistance.
Yet, we must tread carefully. This increased reliance on intelligent systems raises questions about autonomy and the value of human roles. Establishing community conversations on the implications of AGIās presence will smooth the path toward acceptance and constructive integration.
International Collaboration Efforts
When it comes to achieving AGI, collaboration across borders becomes vital. No single country has all the resources or knowledge required to navigate this multifaceted field effectively. Countries must work togetherānot just through competitionāto share knowledge, funding, and strategies. The establishment of international consortiums, akin to the Human Genome Project, could promote open-source AGI initiatives, fostering transparency and innovation.
Points to consider with international collaboration:
- Pooling of Resources:
This can help smaller nations leverage data and technologies that may be out of reach individually. - Standardization of Ethical Guidelines:
Working together can help formulate global norms for AGI development. - Avoidance of Knowledge Silos:
Shared research can prevent isolation and promote a robust intellectual exchange.
"No nation is an island when it comes to AGI; our challenges are global, and so must be our solutions."
The road to AGI is fraught with uncertainties, but understanding these trends shapes the narrative. Anticipating future advancements prepares stakeholders to address challenges head-on, ensuring that AGI evolves responsibly and benefits society as a whole.
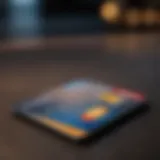
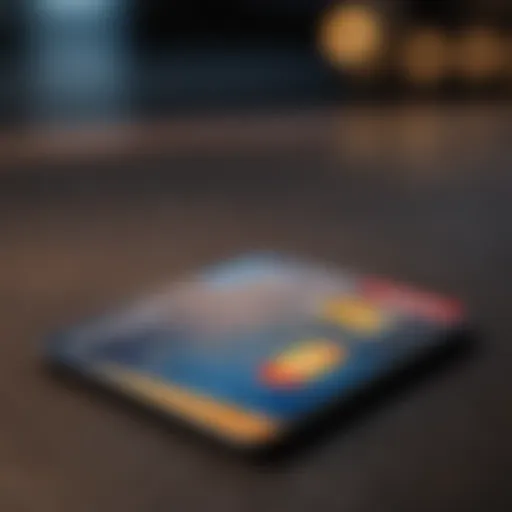